About Our Technology
This AI-powered soft robot leverages deformable materials, soft magnetic grippers, and McKibben pneumatic artificial muscles for unmatched maneuverability, speed, and payload capacity in pipeline inspection. Its machine learning system integrates thermal imaging and gas composition data with a lightweight ensemble deep forest classifier, enhancing leak detection accuracy and adaptability.
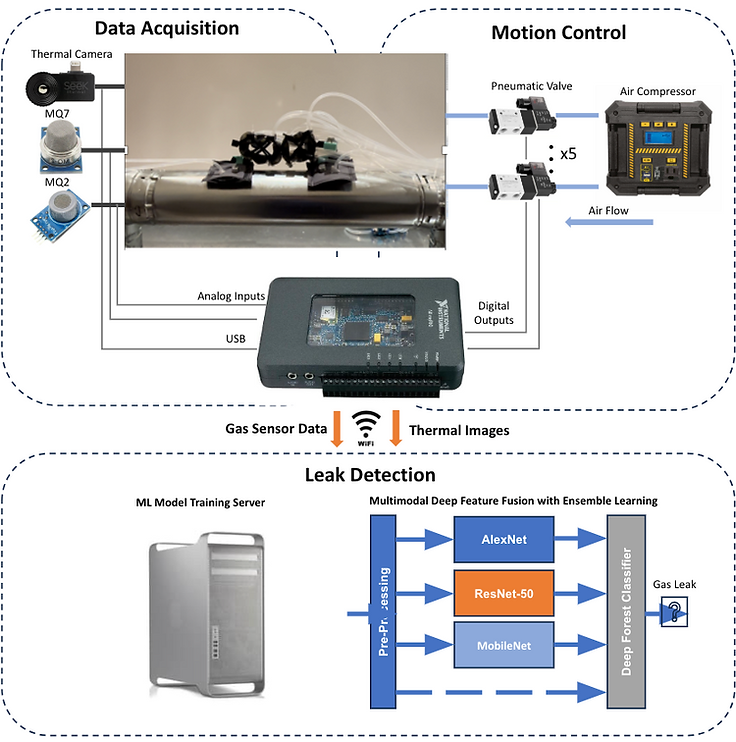
SPIRo Hardware
The hardware portion of our robot innovates upon previous works in the emerging field of soft robotics to create an efficient and adaptable platform for inspecting infrastructure. Due to the complex nature of pipelines and their environments, conventional “rigid” robots are often limited in terms of their movement. Soft robots on the other hand use deformable materials with infinite degrees of freedom for unlimited maneuverability. We employ a custom designed and 3D printed deformable scissor linkage to establish a solid yet deformable structure for the robot. To attach to the piping, we use soft magnetic grippers with origami inspired pouch motors to improve the compactness while still enhancing performance. Finally, we use McKibben pneumatic artificial muscles which have never been used on such robots to improve the maneuverability, speed, and payload capacity of our robot. When crawling along horizontal surfaces, our robot can travel 3 times faster than comparable designs. It also carries double the payload weight of previously reported robots even up vertical surfaces or when turning and maneuvering.
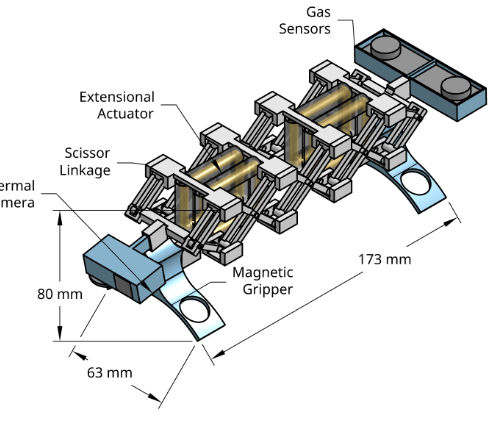
SPIRo In Action
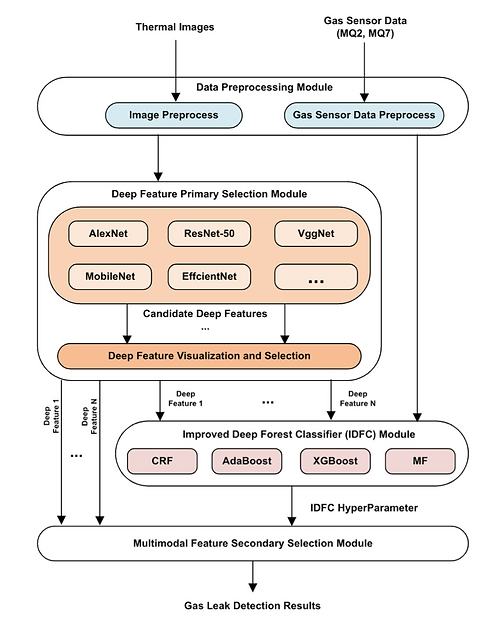
SPIRo Software
The machine learning architecture features two separate modes of data as inputs: thermal images and gas composition data. The gas sensors selected are the analog MQ2 and MQ7 metal oxide sensors, which detect the concentration of methane and carbon monoxide respectively in parts per million. Thermal imaging can be especially helpful in detecting smaller leaks, as methane emitted from gas pipes is heated to a temperature much higher than the surrounding air, making a smaller gas leak easier to detect due to the stark contrast in temperature. However, having multiple modes of data is important since if one sensor were to gather inaccurate data, there would always be a fallback to ensure that leaks are detected. The machine learning architecture developed is also lightweight enough to run on microcontrollers. It consists of an improved ensemble deep forest classifier with a diverse set of base learners, improving diversity and the model’s ability to adapt. To further improve redundancy, multiple convolutional neural networks (CNNs) are implemented to process thermal images, and the feature maps from these CNNs are all fed along with the gas sensor data into the IDFC module. Finally, to provide enhanced interpretability for our models, we use Grad-CAM heatmap overlays.
The SPIRo System
Given the previously defined limitations of current leak detection solutions, we designed SPIRo, a cutting edge AI-based soft robotic system for real-time methane leak detection in upstream pipeline distribution systems. The SPIRo system consists of two parts: the robot SPIRo with built-in sensors and the machine learning framework trained with datasets collected by SPIRo.
SPIRo is an out-pipe crawling robot that navigates along pipelines without disrupting their operation. To accommodate the complex nature of pipelines and the tight underground spaces they are often situated in, SPIRo is designed to be compact, flexible, adaptable, and cost-efficient.
​
Overall, our innovation seeks to detect methane leaks with high reliability and accuracy. Each SPIRo robot can cover 300 miles of pipeline per year; 4300 robots will cover all the pipelines in the U.S. in one year, which may detect up to 85% of fugitive leaks and reduce methane emissions by over 6 million tons annually. One SPIRo system can wirelessly accommodate 6-8 robots working in real-time simultaneously, enlarging the coverage by 6-8 times under the same time span and without human intervention. SPIRo has the potential to prevent millions of tons of super-pollutants from reaching the atmosphere while saving thousands of lives by constantly being on the lookout for dangers.

